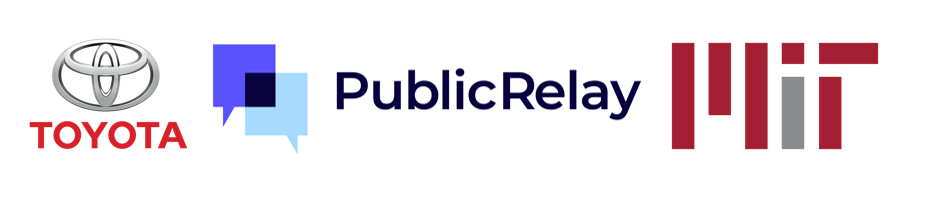
Can technology provide more accurate analysis than humans?
A PublicRelay Partnership with MIT finds that the answer is no.
A study that set human media analysis head-to-head with MIT’s natural language technology processors found that automated solution only had:
- 9% accuracy on detecting key message presence
- 20% accuracy on allocating the correct sentiment
- 33% accuracy on highlighting the precise experience of the customer
Background
Social media analysis is one of the fastest-growing areas of text analytics.
For efficiency and economical reasons, most media analytics providers in the space rely on automated technology to extract emotion and sentiment. But can technology actually provide more accurate text analysis than a human?
In collaboration with Toyota Motor NA Energy & Environmental Research Group, PublicRelay and the intelligent minds at the Massachusetts Institute of Technology (MIT) Analytics Lab set out to find an answer.
The Project
Over the course of three months, the MIT Analytics Lab team tested various technologies to:
- Understand which topics car enthusiasts are discussing in relation to alternate-fuel vehicles on Twitter
- Identify “significant” tweets based on topic inference from above.
The goal was to identify tweets that demand further tracking or direct engagement, and formulate messaging that Toyota might use to drive social media conversations themselves.




The Technology
- MIT built two different modeling approaches to interpret the data– Latent Dirichlet Allocation (LDA) and a Biterm Topic Model (BTM).
- For both processes, the MIT team indicated to the machines which words were topically important.
- The processes would then clean up the tweet text by isolating only the most “important” words and analyze those for key messages, sentiment, and experience according to pre-set definitions.

The Results
- The accuracy of the technological solution versus the human in three key measured attributes was only 9% (key message), 20% (sentiment), and 33% (experience).
- While the technology may be useful in eliminating irrelevant posts before humans analyze them, the MIT team found that 80% of their machine-learning work was simply data clean-up — the computer algorithms had a hard time deciphering commonly used symbols in on Twitter, like @, #, and even text emojis.
- Lastly, the study showed how technology did not handle rapidly changing topics well:
- Analysis over a short time frame fails to detect the quickly‐dissipating influence of one‐off news events and crises.
- Rapidly emerging issues or breaking news such as the Tesla autopilot crash were slow to be reflected in a model.